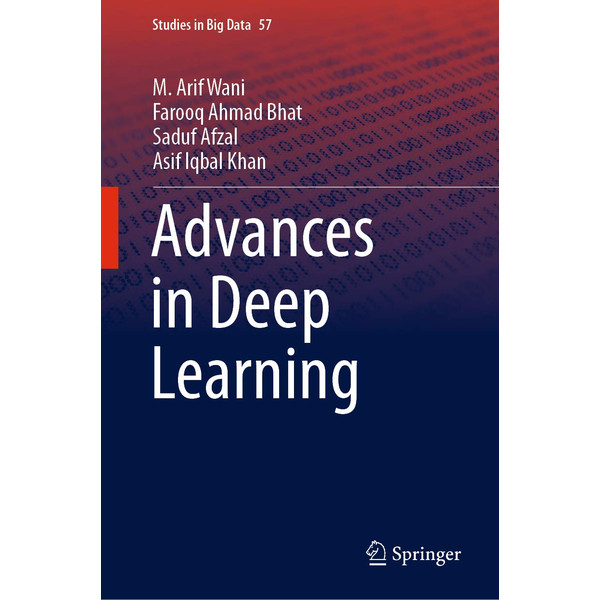

Advances in Deep Learning (Studies in Big Data Book 57) 1st ed. 2020 Edition
About this item
This book introduces readers to both basic and advanced concepts in deep network models. It covers state-of-the-art deep architectures that many researchers are currently using to overcome the limitations of the traditional artificial neural networks. Various deep architecture models and their components are discussed in detail, and subsequently illustrated by algorithms and selected applications. In addition, the book explains in detail the transfer learning approach for faster training of deep models; the approach is also demonstrated on large volumes of fingerprint and face image datasets. In closing, it discusses the unique set of problems and challenges associated with these models.
This book introduces readers to both basic and advanced concepts in deep network models. It covers state-of-the-art deep architectures that many researchers are currently using to overcome the limitations of the traditional artificial neural networks. Various deep architecture models and their components are discussed in detail, and subsequently illustrated by algorithms and selected applications. In addition, the book explains in detail the transfer learning approach for faster training of deep models; the approach is also demonstrated on large volumes of fingerprint and face image datasets. In closing, it discusses the unique set of problems and challenges associated with these models.
Delivery & Return Policy
Exceptions may apply

pdfstore
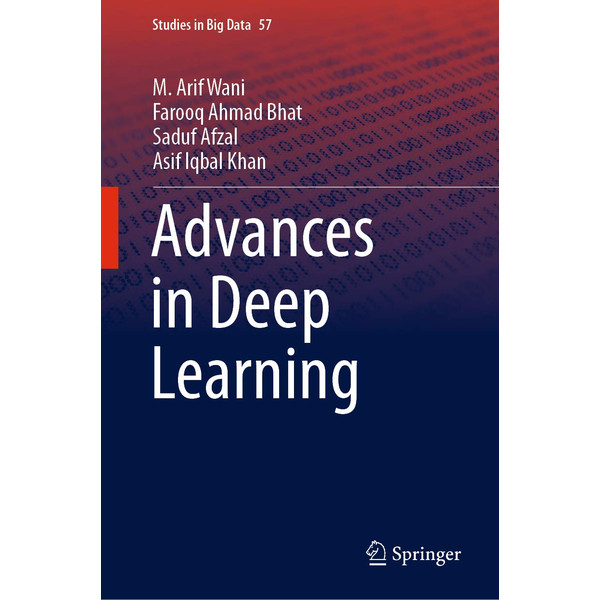

Advances in Deep Learning (Studies in Big Data Book 57) 1st ed. 2020 Edition
Advances in Deep Learning (Studies in Big Data Book 57) 1st ed. 2020 Edition
This book introduces readers to both basic and advanced concepts in deep network models. It covers state-of-the-art deep architectures that many researchers are currently using to overcome the limitations of the traditional artificial neural networks. Various deep architecture models and their components are discussed in detail, and subsequently illustrated by algorithms and selected applications. In addition, the book explains in detail the transfer learning approach for faster training of deep models; the approach is also demonstrated on large volumes of fingerprint and face image datasets. In closing, it discusses the unique set of problems and challenges associated with these models.
This book introduces readers to both basic and advanced concepts in deep network models. It covers state-of-the-art deep architectures that many researchers are currently using to overcome the limitations of the traditional artificial neural networks. Various deep architecture models and their components are discussed in detail, and subsequently illustrated by algorithms and selected applications. In addition, the book explains in detail the transfer learning approach for faster training of deep models; the approach is also demonstrated on large volumes of fingerprint and face image datasets. In closing, it discusses the unique set of problems and challenges associated with these models.